Why your AI project will fail (and how to fix it)
24 August 2020 | 4 min read
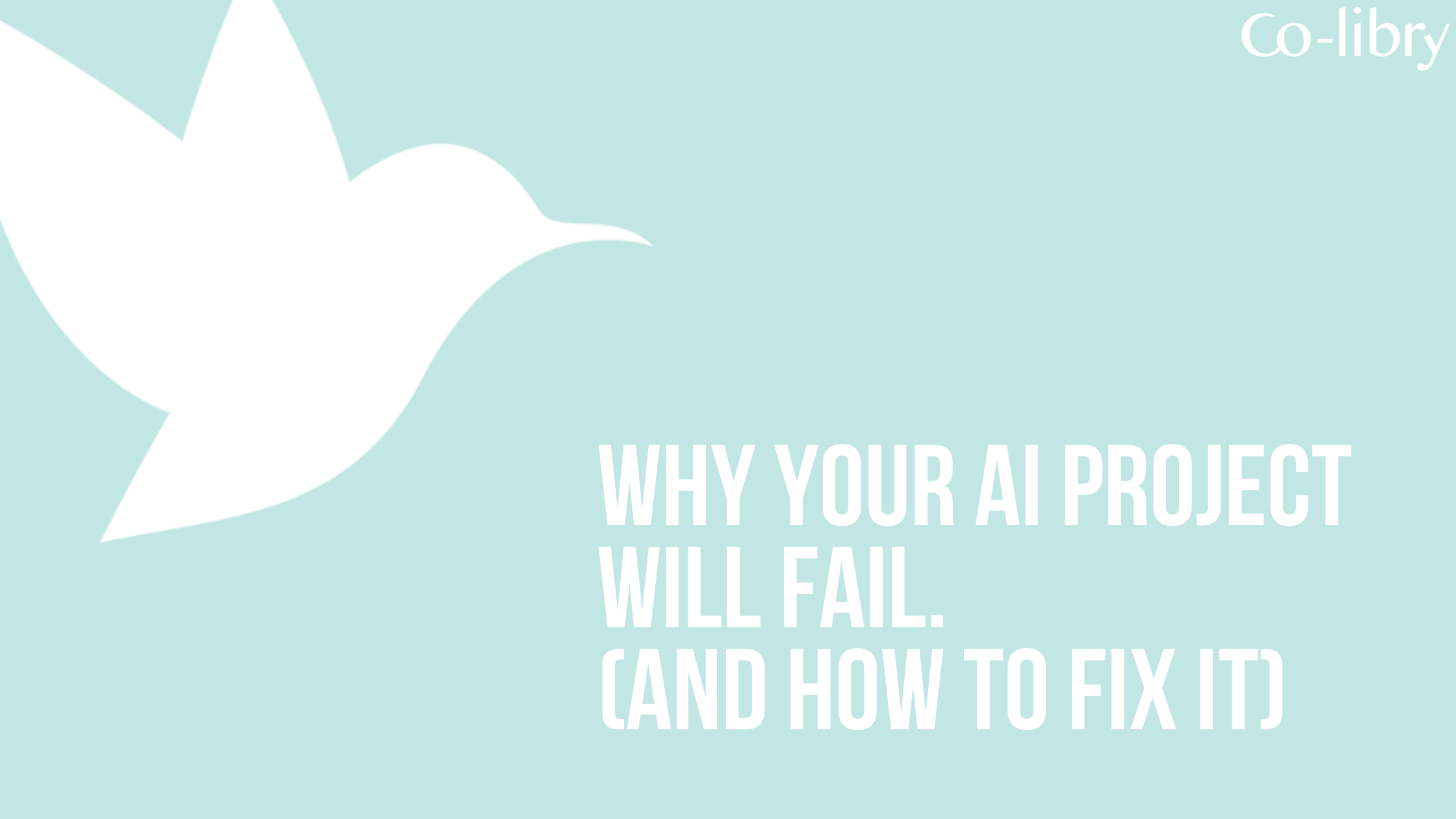
We always hear about Artificial Intelligence being the next big thing for a lot of industries due to the many possibilities that it provides. Yet when we look at the numbers, we see some very worrying statistics. The success rates for many AI and data related projects seem to be hitting an all-time low. And the numbers haven’t improved in almost a decade. But what exactly are companies doing wrong?
First, let’s take a look at the numbers:
- VentureBeat AI reports 87% of data science projects never make it into production
- NewVantage survey reports 77% of businesses report that “business adoption” of big data and AI initiatives continues to represent a big challenge for business.
- Gartner says 80% of analytics insights will not deliver business outcomes through 2022 and 80% of AI projects will “remain alchemy, run by wizards” through 2020.
- A report from Dimensional research states that 8 out of 10 AI projects had failed while 96% ran into problems with data quality, data labelling, and building model confidence.
- 25% of organizations worldwide that are already using AI solutions report up to 50% failure rate according to IDC
Those are some disturbing numbers, right? It seems that a lack of skilled staff and an inability to pinpoint exact customer needs are the main reasons for failure. In this article we will go more in-depth in the reasons why those AI projects are failing and give you tips on how to avoid making the same mistakes. Here are the main reasons why your AI project will probably fail:
- You have poor data quality
- You don’t solve the right problems
- General e-commerce solutions don’t work for jobs, real estate, and car websites.
- You don’t have enough data scientists
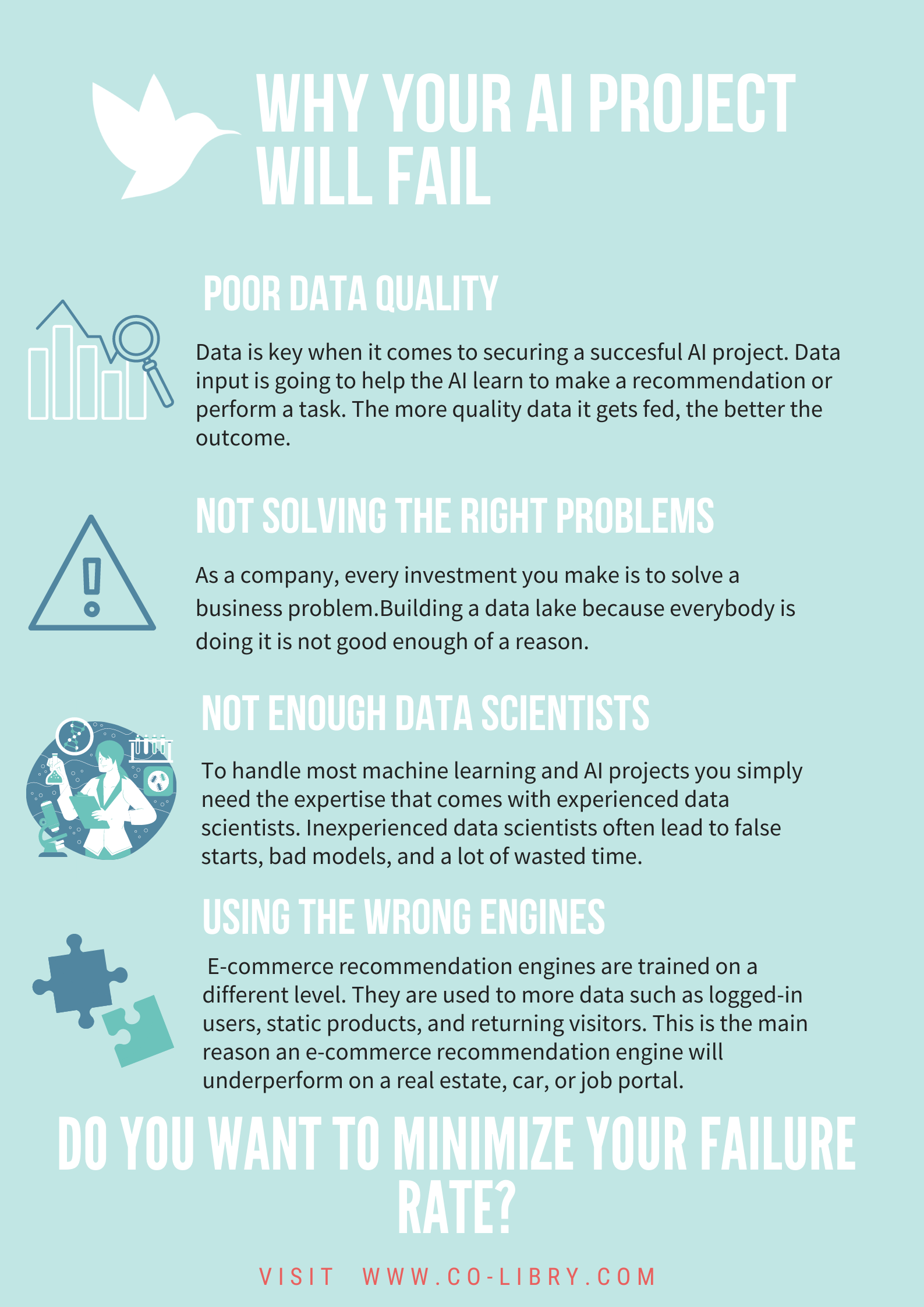
1. You have poor data quality
Data is key for AI to become successful. One of the best analogies is that a car can not drive without fuel. A car is made out of amazing technology but still needs the right fuel to be able to drive. The same can be said about AI. In order to teach AI to perform a specific task or a series of tasks, it must have data. That data input is going to help the AI learn to make a recommendation or perform a task. The more quality data it gets fed, the better the outcome.
An example of what could happen when you don’t prepare your data well: A study by Trifacta shows that 59 percent of data inaccuracy results from miscalculating demand and 26 percent from targeting the wrong prospects.
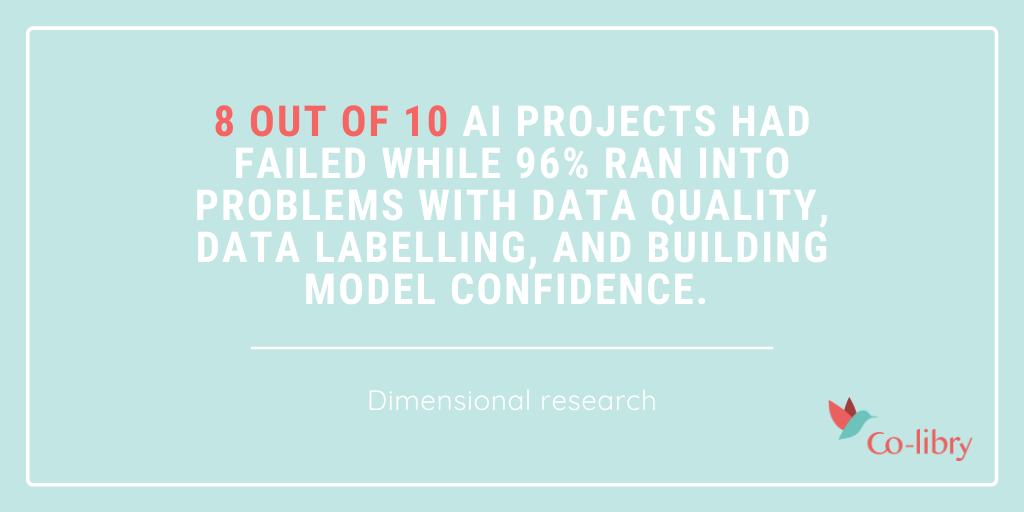
2. You don’t solve the right problems
As a company, every investment you make is to solve a business problem.
Building a data lake because everybody is doing it is not good enough of a reason. furthermore, “Everybody is doing it” is not a good enough reason to do anything, and certainly not to start dabbling with Artificial Intelligence.
Of course, you can let your developers and data scientists play in a sandbox with the data to learn and gain expertise. But if they continue to come up with amazing solutions that do not solve important business problems, then you’re probably doing something wrong.
Data scientists can suggest potential solutions, but the problem statement and enterprise knowledge should always be coming from the business.
3. General e-commerce solutions don’t work for jobs, real estate, and car websites.
A common mistake is that a lot of companies just try to implement general e-commerce solutions, just because they are available in masses and seem easy enough to implement. Yet E-commerce solutions, just like the name suggests, will only work on e-commerces.
E-commerce recommendation engines are trained on a different level. They are used to more data such as logged-in users, static products, and returning visitors. This is the main reason an e-commerce recommendation engine will underperform on a real estate, car, or job portal.
For real estate, car, and job portals, the recommendation engine needs to adapt constantly, because the listings on those portals change all the time. According to Zillow, the average time it takes to sell a house is 65 to 93 days. On an e-commerce website, the products stay online for a much longer period and the website doesn’t have to change every couple of weeks.
The second difference is the way visitors interact with the website. Visitors usually only need to do one ‘purchase’ on a real estate, car, or job portal. After that, they will not return, at least not for a very long time. This makes it more difficult to create clusters and profiles because the people in those profiles are constantly changing.
4. You don’t have enough data scientists
It seems that today just about anyone who has ever worked in data analytics is labeling themselves a data scientists after taking an online masterclass. The reality is that in order to handle most machine learning and AI projects you simply need the expertise that comes with experienced data scientists. Inexperienced data scientists often lead to false starts, bad models that look good at first, and a lot of wasted time.
But hiring data scientists is a tricky process in today’s current economic environment. These highly-trained resources are scarce and very expensive. And for good reason: data science is a complex occupation that requires years of math, statistics, and programming skills to become proficient.
“No matter how good the team or how efficient the methodology, if we’re not solving the right problem, the project fails.” – Woody Williams
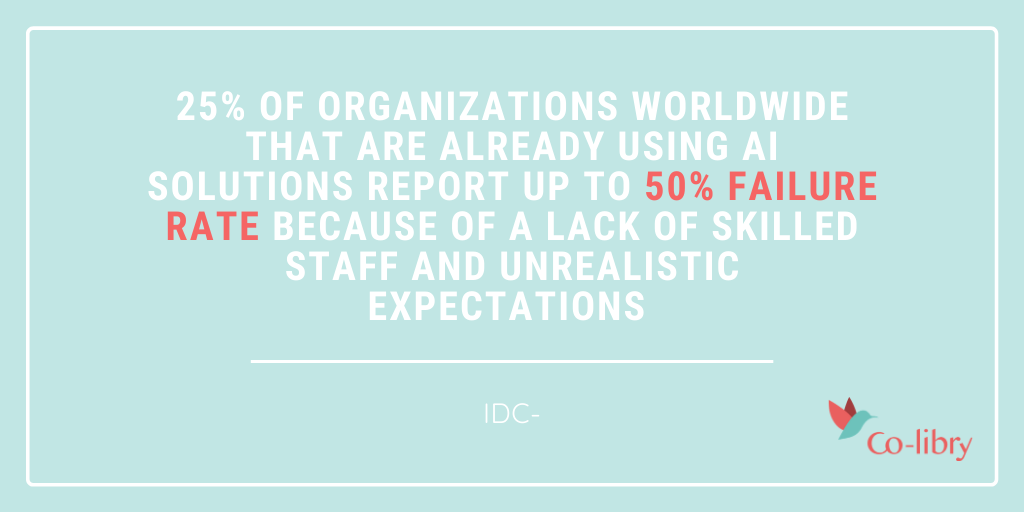
How to minimize your failure rate?
There is also some good news. Now that we know what the common pitfalls are we can do something about it. So how exactly do you avoid those problems and minimize your failure rate?
Capture high-quality data
It all starts with the right data. It is without a doubt the most important factor in order to be successful with AI. Even tho data preparation is a timeconsuming and often inefficient process, it’s vital to the success of every AI project.
Solve the right problem
Always start with the problem you want to solve and what solutions your business is really looking for. It’s also helpful to set up your expectations in terms of revenue and what impact AI will have on your company.
Decide whether you want to build your AI inhouse or not
If you want to build the AI solutions yourself, you need to determine if the investment of time, effort, and capital will provide you with meaningful ROI. Because if it does not, you should not go down this path.
Many companies are attempting to build their own internal AI teams in-house. This takes significant time and capital with no guarantees of success.
Of course, there is also an option to use Off-the-shelf AI solutions, but they are often only made for e-commerce stores. They do not work for real estate, car, or job websites and they either need extensive customization to bring value or they simply never bring value at all.
At Co-libry, our approach of offering Plug-and-play AI solutions enables you with world-class AI algorithms without the time and risk of building an in-house team.
References
https://www.businesswire.com/news/home/20190708005039/en/
https://blogs.gartner.com/andrew_white/2019/01/03/our-top-data-and-analytics-predicts-for-2019/
http://newvantage.com/wp-content/uploads/2018/12/Big-Data-Executive-Survey-2019-Findings.pdf
https://venturebeat.com/2019/07/19/why-do-87-of-data-science-projects-never-make-it-into-production/
https://betanews.com/2020/01/23/data-confidence-ai-failures/
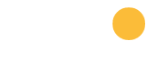
AI and personalization made easy
Schedule your call today!
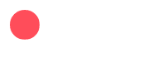
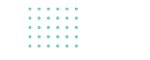
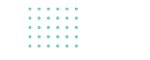
AI and personalization made easy
Schedule your call today!
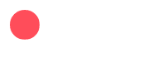
How House Alerts Help To Obtain Higher Click-Through Rates
Thanks to house alert systems, you can quickly inform potential buyers when new properties that match their criteria show up. Here is a step-by-step guide that will show you how you can retarget potential customers and help them find the house of their dreams.
Two Unique Dashboards That Will Help Your Real Estate Agents Close More Deals
Being successful in sales is about knowing your product inside out and knowing everything there is to know about your customer. Luckily, it’s easier to achieve these two goals today than ever before by using some unique dashboards. Read everything about it here:
How Artificial Intelligence is Shaping the Online Real Estate Market
Now in 2021, the message is clear; AI is here, and it’s here to stay. But what impact does this technology have on the real estate market? Read everything about it in this article